OPHTHALMOLOGY
Obtain Your Labeled Eye Datasets From Medrays
We are proud to offer a wide range of high-quality labeled eye datasets designed to support the development of machine learning models in the field of ophthalmology. Our datasets are meticulously curated and annotated by a team of experienced labelers, guaranteeing the accuracy and reliability of the provided labels. By leveraging our labeled datasets, researchers and developers can expedite their advancements in AI-driven ophthalmology and make significant contributions to improving patient care outcomes. At Medrays, we place utmost importance on data privacy and security, implementing robust measures to protect sensitive information and ensuring compliance with regulatory standards. Our dedicated team is committed to delivering exceptional customer support, ensuring a seamless experience as you access and utilize our labeled eye datasets. Choose Medrays as your trusted partner for reliable and high-quality eye datasets that drive innovation and pave the way for breakthroughs in the field of eye care.
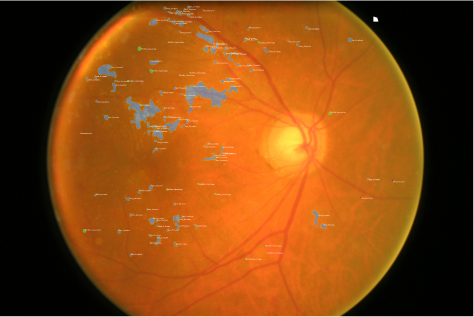
Why Precisely Labeled Eye Datasets Are Critical in Ophthalmology?
Accurate and reliable labeling of datasets is crucial for the development of highly effective artificial intelligence (AI) models in ophthalmology. The precision and accuracy of AI models are greatly influenced by the quality of the labeled datasets used for training. By ensuring accurate labeling, we enable AI models to learn intricate patterns, abnormalities, and features within the eye, leading to improved diagnostic capabilities. Our perfectly labeled eye datasets enable AI models to classify and segment different regions of the eye with precision, including the optic nerve, retina, macula, and blood vessels. This level of detail facilitates the detection of various eye conditions, such as diabetic retinopathy, glaucoma, macular degeneration, and cataracts, enabling early detection and timely intervention. Moreover, precise labeling ensures consistency across different samples, eliminating potential ambiguities or misinterpretations. It establishes a standardized framework for training and evaluating AI models, enhancing their reliability and reproducibility. When deployed in clinical settings, accurate and consistent results build trust and confidence among healthcare professionals, ultimately improving patient care outcomes.
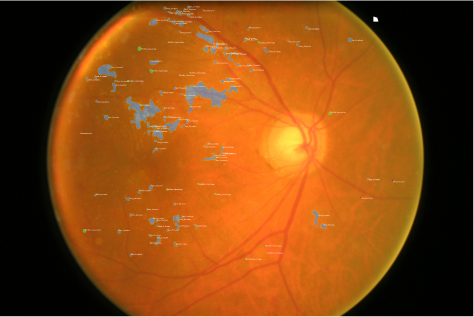
Image Classification
Image classification involves labeling images of the eye to classify them into different categories based on their features, such as the presence of certain diseases or conditions. By accurately labeling images, AI models can learn to identify and distinguish between various eye conditions, such as diabetic retinopathy, macular degeneration, or glaucoma. This enables ophthalmologists to efficiently assess and prioritize cases, providing timely and appropriate interventions for patients.
Object Detection
Object detection in ophthalmology entails labeling objects or specific features within an image, such as blood vessels or optic nerve heads. With precisely labeled datasets, AI models can accurately locate and identify these objects, assisting in the detection of abnormalities or pathologies. For example, by accurately detecting and measuring the diameter of blood vessels, AI models can aid in the early diagnosis of conditions like hypertensive retinopathy or diabetic retinopathy.
Segmentation
Segmentation involves labeling specific regions or structures within an image, such as the retina or optic nerve. Precisely labeled datasets are essential for training AI models to accurately segment these regions, enabling detailed analysis and evaluation. For instance, by segmenting the retina, AI models can assist in quantifying the thickness of retinal layers, identifying areas of thinning or thickening that may indicate the progression of retinal diseases.
Optical Coherence Tomography (OCT) Labeling
OCT labeling focuses on segmenting and classifying features within OCT images of the eye, such as the retinal layers, optic nerve head, or choroid. Precisely labeled datasets are crucial for training AI models to identify specific structures and anomalies within OCT scans. For example, by accurately labeling the retinal layers, AI models can aid in detecting changes in thickness or identifying specific layers affected by diseases like macular edema or retinal detachment.
Fundus Image Labeling
Fundus image labeling involves labeling features within fundus images of the eye, such as the optic disc or blood vessels. Precisely labeled datasets allow AI models to identify and analyze specific structures within fundus images. For instance, by accurately labeling the optic disc, AI models can assist in measuring its size, detecting abnormalities like optic disc drusen or optic disc swelling, and monitoring changes over time.
Histopathology Image Labeling
Histopathology image labeling focuses on labeling features within histopathology images of the eye, such as the cornea, iris, or retina. Precisely labeled datasets enable AI models to identify and analyze microscopic structures within these images. For example, by accurately labeling the different layers of the cornea, AI models can assist in diagnosing corneal disorders or evaluating the extent of corneal damage in conditions like keratoconus or corneal dystrophy.
Enhance Your Ophthalmology AI Models With Our Accurate Data Labeling
Frequently Asked Questions
1.
Why is data labeling important in ophthalmology?
Data labeling is important in ophthalmology as it allows for the training of AI models to diagnose eye conditions and assist in treatment planning, improving the accuracy and efficiency of ophthalmic care.
2.
How is ophthalmology data labeling done?
Ophthalmology data labeling is typically performed by trained medical labelers who possess the necessary expertise in interpreting ophthalmic images and identifying specific features and abnormalities.
3.
What types of data are labeled in ophthalmology?
In the field of ophthalmology data labelling, various types of data are annotated. These include retinal images, optic nerve images, corneal topography maps, and other ocular imaging modalities. Additionally, important features such as anatomical structures, lesions, abnormalities, and other relevant aspects are also marked and identified during the labelling process.
4.
Who performs ophthalmology data labeling?
With the assistance of skilled medical professionals, data labeling tasks in ophthalmology are typically performed by trained medical labelers who possess expertise in the field. These labelers specialize in ophthalmology and are well-equipped to provide accurate and clinically relevant annotations.
5.
What are the common annotation techniques used in ophthalmology data labeling?
Common annotation techniques used in ophthalmology data labeling include segmentation, where anatomical structures such as the optic disc or retinal vessels are outlined, classification, where images are labeled as normal or with specific pathologies, and localization, where the precise location of abnormalities or lesions within an image is identified.