Natural Language Processing
NLP Data Labeling: The Power of Language for AI Models
Transform medical language into insights with our NLP data labeling services! Our dedicated team of experts is committed to delivering precise and efficient annotation solutions tailored to your specific needs in the medical industry. With expertise in various essential tasks for medical NLP applications, such as named entity recognition (NER), relation extraction, sentiment analysis, and text classification, we ensure accurate labeling of diseases, symptoms, treatments, and more. By leveraging our NLP data labeling services, you can propel the advancement of AI models in medical applications, extracting valuable information from medical texts to enhance diagnosis, treatment, and overall patient care. Our specialized annotation services enable the identification of crucial relationships between medical entities, analysis of patient sentiment for improved feedback and reviews, and effective categorization of medical documents for easy retrieval and analysis.
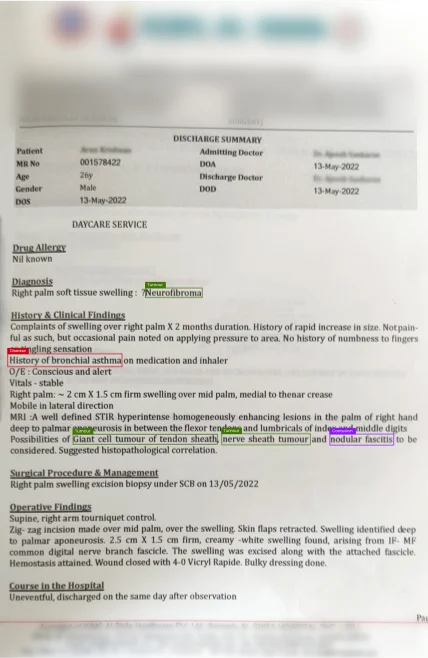
As Always Accuracy is the Key
At Medrays, accuracy is not just a goal; it's our foundation. We strive to provide data labeling services that meet the highest standards of precision and reliability. With our commitment to accuracy and our comprehensive understanding of the medical domain, you can trust us to optimize your NLP models for success, ensuring that they deliver the most accurate, reliable, and equitable results in healthcare applications. In the medical industry, the accuracy and reliability of Natural Language Processing (NLP) models are vital for patient safety and effective clinical decision-making. To achieve high-performing AI models, quality data labeling plays a crucial role in training them with consistent and reliable data, resulting in more precise and effective language-based predictions. In the medical field, accurate data labeling is particularly significant as it helps mitigate bias that can arise from variations in language use and cultural context. By ensuring precise and reliable labeling, we can optimize NLP models to deliver accurate and equitable results across diverse patient populations, ultimately improving healthcare quality and outcomes. Our dedicated team understands the criticality of accuracy and is fully committed to providing data labeling services of the highest precision and quality. We recognize that even minor errors or inconsistencies in data labeling can have significant consequences in the medical domain. Hence, we adopt a meticulous approach, leveraging our expertise and industry knowledge to ensure the accuracy of every annotation. Through thorough analysis, rigorous quality control measures, and adherence to industry standards, we guarantee that your NLP models are trained on trustworthy and reliable data. Additionally, our team is well-versed in the unique challenges of medical language and terminology. We understand the importance of capturing the intricacies and nuances of medical texts to enable accurate interpretation and analysis. By accurately labeling medical entities, relationships, sentiments, and document categories, we empower your NLP models to extract meaningful insights and support healthcare professionals in making informed decisions.
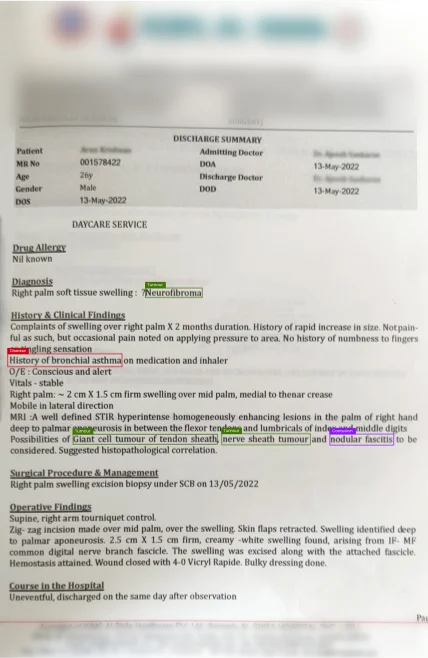
Named Entity Recognition (NER) Labeling
We label specific entities in unstructured data, including diseases, symptoms, treatments, drugs, and other medical terms. This precise and detailed annotation ensures that NLP models can accurately identify and extract relevant information, leading to enhanced medical analysis and decision-making.
Relation Extraction Labeling
We annotate the relationships between entities in the text. This includes capturing connections such as the association between a symptom and a disease or the correlation between a drug and its side effects. The labeled relationships provide valuable insights for medical research, treatment planning, and optimizing patient care.
Sentiment Analysis Labeling
We accurately label the sentiment or emotion expressed in the text. This includes categorizing patient reviews as positive, negative, or neutral, allowing healthcare providers to gauge patient satisfaction levels, identify areas for improvement, and tailor their services accordingly. Our sentiment analysis labeling empowers AI models to better understand and respond to patient feedback.
Text Classification Labeling
Our team excels in classifying unstructured data into predefined categories relevant to the medical domain. Whether it's categorizing medical documents based on specialties, topics, or formats, our meticulous labeling ensures easy organization and retrieval of information. This supports efficient research, knowledge management, and informed decision-making for healthcare professionals.
Intent Detection Labeling
We specialize in accurately labeling the intent or purpose behind textual data. By identifying whether a patient is requesting an appointment, seeking medical advice, or expressing a concern, we enable AI models to automate processes, improve response times, and streamline communication. Our intent detection labeling facilitates efficient and personalized interactions between patients and healthcare providers.
Emotion Detection Labeling
We identify and label the emotions or feelings expressed in the text. This includes capturing a range of emotions such as anxiety, frustration, happiness, or relief. Emotion detection labeling enhances the understanding of patient experiences, enables personalized care approaches, and supports mental health assessment and interventions.
Unlock The Full Potential Of Your NLP Projects With Our Expert Data Labeling Services
Frequently Asked Questions
1.
Why is data labeling important in NLP?
Data labeling is crucial in NLP as it provides labeled examples that serve as training data for machine learning models, allowing them to understand and process human language accurately. As a result, models can perform better in tasks like sentiment analysis, language translation, and text classification.
2.
How is NLP data labeling done?
Our expert annotators, assisted by skilled medical professionals, perform NLP data labeling. These annotators review and annotate text data following predefined guidelines or criteria, which may include labeling sentiment, identifying named entities, or classifying text into specific categories.
3.
What types of data are labeled in NLP?
Various types of data are labeled in NLP, including text snippets, sentences, or entire documents. Labels may include sentiment categories (positive, negative, neutral), named entities (person names, locations), part-of-speech tags, or specific categories for text classification tasks.
4.
How accurate is NLP data labeling?
At Medrays, we place a strong emphasis on accuracy in NLP data labeling. We have implemented robust quality control measures and stringent guidelines to ensure high precision in our annotations. Through a combination of experienced annotators, comprehensive training, and meticulous review processes, we strive to provide highly accurate labeled data for NLP tasks.
5.
What are the benefits of using labeled NLP data in research or NLP applications?
Using labeled NLP data in research or NLP applications offers several benefits, such as improved model performance and generalization, faster development of NLP models, benchmarking and comparison of different models, and facilitating the understanding and interpretation of natural language phenomena. Additionally, labeled data allows for the evaluation and validation of NLP algorithms and techniques.